Product Code: ICA13_2008
System Identification and Height Control of Laser Cladding Using Adaptive Neuro-Fuzzy Inference Systems
Authors:
Mohammad Farshidianfar, University of Waterloo; Waterloo USA
Amir Khajepour, University of Waterloo; Waterloo ON Canada
Meysar Zeinali, University of Laurentian; Sudbury ON Canada
Adrian Gerlich, University of Waterloo; Waterloo ON Canada
Presented at ICALEO 2013
Laser cladding is an emerging technology which has recently received a significant attention by researchers due to its promising manufacturing features. A complicated object can be fabricated directly from a CAD model by means of a layer-by-layer manufacturing using this technique. In many physical processes it is very difficult or even impossible to develop an analytical model due to process complexities. In the laser cladding process, there is a complex interaction between heat conduction, convection, radiation and mass transfer that makes it difficult to model the process analytically. On the other hand numerical modeling requires cumbersome calculations which are not suitable for real-time control applications. Thus, producing a clad with desired mechanical, metallurgical and geometrical properties is a hard task to achieve in the laser cladding process. Clad height is one of the main important outputs of process which is very crucial in applications such as rapid prototyping and coating. However because of the obstacles mentioned above the identification and control of the clad height is hard to achieve.
Adaptive neuro-fuzzy inference systems (ANFIS) are utilized to identify and control the clad height in the laser cladding process. The scanning speed of the substrate is used as the control action in the closed-loop controller. The feedback signal is obtained by a CCD camera using a 1.1 kW fiber laser and a 5-axis CNC machine. Several experiments are carried out in order to obtain a set of training data for the learning process of the ANFIS networks. First, the process is identified by means of an ANFIS network through a hybrid learning algorithm. The inverse dynamics of the ANFIS plant is later obtained in an ANFIS inverse learning scheme. The inverse dynamics is used in a neuro-fuzzy structure in order to obtain an ANFIS controller for the process. A complete control system is designed by tuning the ANFIS controller as a combined unit. Results of the controller are experimentally verified. Satisfactory results are obtained both in process modeling and process control.
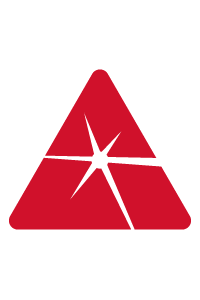
$28.00
Members: $28.00
Note: When applicable, multiple quantity discounts are applied once the items are added to your cart.